Lawrence D. Brown
Distinguished Lecture Series
PhD Students’ Best Papers Award
In honor of the late Professor Lawrence D. Brown, a distinguished member of the Wharton School's Statistics and Data Science Department, The Wharton School, together with the department, established the Lawrence D. Brown Distinguished Lecture Series and PhD Students’ Best Papers Award in 2021. These events celebrate Professor Brown's legacy and foster academic excellence among PhD students.
Department of Statistics and Data Science
The Wharton School
University of Pennsylvania
Lawrence D. Brown
Lawrence David Brown, the Miers Busch Professor of Statistics at the Wharton School, passed away on February 21, 2018, at the age of 77. Dr. Brown was a prolific researcher, publishing five books and more than 170 journal articles. His pioneering work in statistical decision theory and nonparametric function estimation, among other areas, has left an indelible mark on the field. His contributions extended to sequential analysis, foundations of statistical inference, properties of exponential families, interval estimation, bioequivalence, and analysis of census and call center data.
A member of the National Academy of Sciences and the American Academy of Arts and Sciences, Dr. Brown received numerous honors, including an honorary Doctor of Science degree from Purdue University in 1993, the Wilks Memorial Award from the American Statistical Association in 2002, and the C. R. and B. Rao Prize in 2007. His professional service included serving as president of the Institute of Mathematical Statistics, co-editing The Annals of Statistics, and chairing the NRC Committee on National Statistics from 2010 until his passing in 2018.
Professor Brown was deeply committed to teaching and mentoring, having supervised 37 PhD students, many of whom have become prominent figures in the field of statistics both in the United States and internationally. In 2011, he received the Provost’s Award for Distinguished PhD Teaching and Mentoring, a testament to his dedication.
Born on December 16, 1940, Dr. Brown graduated from the California Institute of Technology in 1961 and earned his PhD in mathematics from Cornell University in 1964. Before joining the faculty at the University of Pennsylvania, he held positions at the University of California, Berkeley; Cornell University; and Rutgers University.
Annual Events
Each November, the Statistics and Data Science Department hosts two main events over the course of one week to honor Professor Brown’s legacy:
I. Distinguished Lecture Series
A committee of three members selects a prominent speaker from the international statistics and data science community to deliver two to three lectures. These lectures bring cutting-edge research and insights to the department and the broader academic community.
II. Best PhD Student Paper Awards
PhD students within the department are invited to submit their papers for consideration. A committee reviews the submissions and selects papers to be presented. From these presentations, the committee awards a prize to the best paper(s), funded by the Brown family. This award acknowledges outstanding research contributions from emerging scholars in the field of statistics.
IMS Lawrence D. Brown PhD Student Award
Additionally, the Institute of Mathematical Statistics (IMS) offers the IMS Lawrence D. Brown PhD Student Award on a larger scale, which is awarded annually to recognize exemplary PhD student work in statistics. For more information on this award, please visit the IMS website.
Distinguished Lecture Series
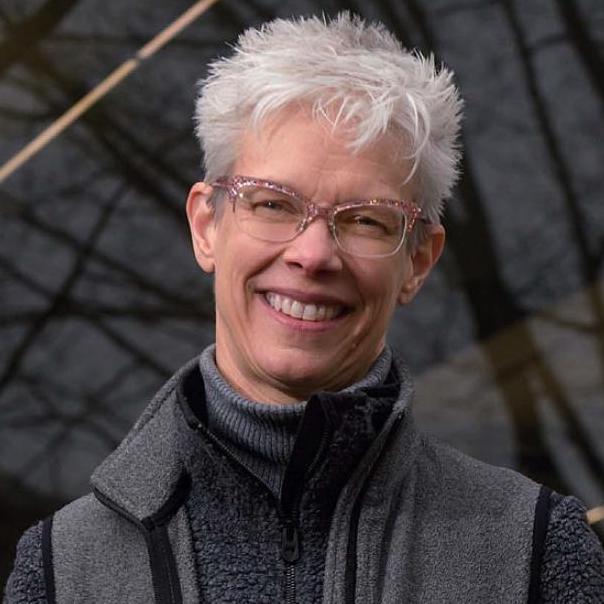
Susan Murphy
Harvard University
Susan A. Murphy is Mallinckrodt Professor of Statistics and of Computer Science and Associate Faculty at the Kempner Institute, Harvard University. Her research focuses on improving sequential decision making via the development of online, real-time reinforcement learning algorithms. Her lab is involved in multiple deployments of these algorithms in digital health. She is a member of the US National Academy of Sciences and of the US National Academy of Medicine. In 2013 she was awarded a MacArthur Fellowship for her work on experimental designs to inform sequential decision making. She is a Fellow of the College on Problems in Drug Dependence, Past-President of Institute of Mathematical Statistics, Past-President of the Bernoulli Society and a former editor of the Annals of Statistics.
November 19, 2024
In this talk I will discuss first solutions to some of the challenges we face in developing online RL algorithms for use in digital health interventions targeting patients struggling with health problems such as substance misuse, hypertension and bone marrow transplantation. Digital health raises a number of challenges to the RL community including different sets of actions, each set intended to impact patients over a different time scale; the need to learn both within an implementation and between implementations of the RL algorithm; noisy environments and a lack of mechanistic models. In all of these settings the online line algorithm must be stable and autonomous. Despite these challenges, RL, with careful initialization, with careful management of bias/variance tradeoff and by close collaboration with health scientists can be successful. We can make an impact!
November 20, 2024
Adaptive sampling methods, such as reinforcement learning (RL) and bandit algorithms, are increasingly used for the real-time personalization of interventions in digital applications like mobile health and education. As a result, there is a need to be able to use the resulting adaptively collected user data to address a variety of inferential questions, including questions about time-varying causal effects. However, current methods for statistical inference on such data (a) make strong assumptions regarding the environment dynamics, e.g., assume the longitudinal data follows a Markovian process, or (b) require data to be collected with one adaptive sampling algorithm per user, which excludes algorithms that learn to select actions using data collected from multiple users. These are major obstacles preventing the use of adaptive sampling algorithms more widely in practice. In this work, we proved statistical inference for the common Z-estimator based on adaptively sampled data. The inference is valid even when observations are non-stationary and highly dependent over time, and (b) allow the online adaptive sampling algorithm to learn using the data of all users. Furthermore, our inference method is robust to miss-specification of the reward models used by the adaptive sampling algorithm. This work is motivated by our work in designing the Oralytics oral health clinical trial in which an RL adaptive sampling algorithm is used to select treatments, yet valid statistical inference is essential for conducting primary data analyses after the trial is over.
November 21, 2024
Adaptive treatment assignment algorithms, such as bandit and reinforcement learning algorithms, are increasingly used in digital health interventions. Between implementation of the digital health intervention, data analyses are critical for producing generalizable knowledge and deciding how to update the intervention for the next implementation. However the replicability of these between-implementation data analyses has received relatively little attention. This work investigates the replicability of statistical analyses from data collected by adaptive treatment assignment algorithms. We demonstrate that many standard statistical estimators can be inconsistent and fail to be replicable across repetitions of the clinical trial, even as the sample size grows large. We show that this non-replicability is intimately related to properties of the adaptive algorithm itself. We introduce a formal definition of a 'replicable bandit algorithm' and prove that under such algorithms, a wide variety of common statistical analyses are guaranteed to be consistent. Our findings underscore the importance of designing adaptive algorithms with replicability in mind, especially for settings like digital health where deployment decisions rely heavily on replicated evidence. We conclude by discussing open questions on the connections between algorithm design, statistical inference, and experimental replicability.
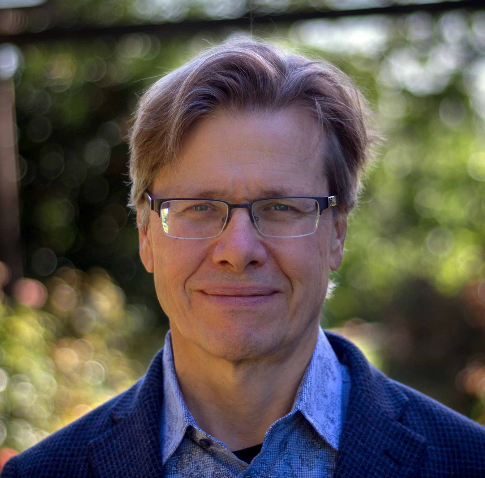
David Donoho
Stanford University
David Donoho has studied the exploitation of sparse signals in signal recovery, including for denoising, superresolution, and solution of underdetermined equations. His research with collaborators showed that ell-1 penalization was an effective and even optimal way to exploit sparsity of the object to be recovered. He coined the notion of compressed sensing which has impacted many scientific and technical fields, including magnetic resonance imaging in medicine, where it has been implemented in FDA-approved medical imaging protocols and is already used in millions of actual patient MRIs.
In recent years David and his postdocs and students have been studying large-scale covariance matrix estimation, large-scale matrix denoising, detection of rare and weak signals among many pure noise non-signals, compressed sensing and related scientific imaging problems, and most recently, empirical deep learning.
April 23, 2023
A conventional narrative tells us that Data Science is just a rebranding of traditional statistics. This talk explores the idea that there is a “Data Science” mindset derived from modern digital life, and a “Statistics Mindset” derived from long intellectual tradition. These mindsets breed two completely different mental realities ie. different thoughts we can hold in mind and pay attention to. Because of this, there is a gaping divide between what residents of each mental reality can focus upon, produce and value. Downstream of this, two completely separate discourses and cultures are developing.
In our view, the two sides don’t properly understand that there are two sides, and that severe challenges are caused by this split. This shows up when each camp reflects on the other in frequent frustration, pointless conversations and negative emotions. This can be seen in situations where teams from each side of the divide both do research about the same topic, and try to engage with each other's research.
It is important for the statistics tradition to drop the blinders and see the situation clearly, to finally benefit from the existence of the data science reality. Similarly, data science could make better progress by clearly understanding what the statistics tradition can offer.
Finally, the biggest opportunities will come for those who can become bicultural. The talk should be accessible to a broader audience. This is joint work with Matan Gavish (Hebrew University CS).
April 24, 2023
Modern ML systems are extraordinarily data hungry; and some major commercial players are said to now be using synthetic data to train their most ambitious ML systems. Also, AI-generated data will soon flood the internet, perhaps to the point where most available data are synthetic. Recently ML research has started to confront the larger issues that synthetic data might pose, including a future where most or all of the data available for an ML training are synthetic. A number of ML papers became prominent after promoting the idea of “model collapse”, the “curse of recursion”, “model autophagy disorder”. Featuring experiments and some very basic theoretical argumentation they promoted a storyline where successive recycling of purely synthetic data led to model degeneration.
In contrast, Mathematical Scientists have looked at the same setting as the ML researchers, and developed a more balanced view of the situation, depending on the synthetic data use case, no such collapse occurs. Empirical work with canonical LLMs and diffusion models confirms the absence of collapse, in the recommended use case.
I will review the setting, the narrative promoting to panic and counter narratives leading to a calmer view. The talk should be accessible to a broader audience, as most of the research in this area consists of analysis at the statistics undergraduate major or master’s level — although one could transplant the basic questions into fancier settings if one liked.
This is joint work with Apratim Dey of Stanford Statistics and a CS team at Stanford.
April 25, 2023
We review some Compressed Sensing theory through the lens of Approximate Message Passing, and minimax decision theory concerning shrinkage estimates. AMP powers up a simple estimator for an elementary problem into a procedure for a drastically more ambitious problem of compressed sensing. In this case the simple estimator is James-Stein shrinkage and we use it to construct a procedure of multiple measurement vector compressed sensing.
We discuss the State Evolution analysis of this procedure, prove and empirically verify all the predictions of state evolution, and so on. We discuss the state evolution theory of Compressed Sensing in the sense of sparsity-undersampling phase diagram. In the large dimensional limit both in system size and vector size, with Gaussian sensing matrices, James-Stein has a theoretically unimprovable phase diagram and empirically works near-optimally even in low vector dimensions. In particular this is far better than the convex optimization approaches.
This is joint work with Apratim Dey (Stanford Statistics).
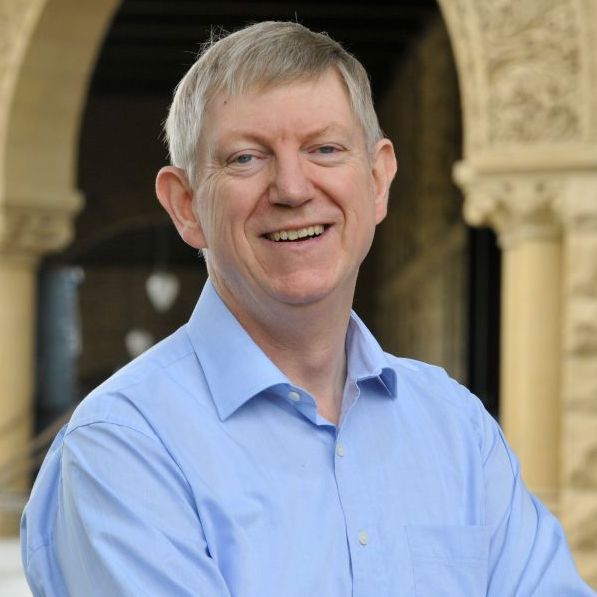
Iain Johnstone
Stanford University
Dr. Johnstone is a statistician with research interests in statistical decision theory and wavelet-like methods (and their uses) in estimation theory, asymptotics and application areas such as statistical inverse problems and statistical signal processing. Other interests include simulation methodology, volume tests of significance, hazard rate estimation and maximum entropy methods.
November 16, 2022
When data is high dimensional, widely used multivariate methods such as principal component analysis can behave in unexpected ways. Upward bias in sample eigenvalues and inconsistency of sample eigenvectors are among the new phenomena that appear. In this expository overview talk, I will try to use (amateur!) graphics and heuristic arguments to explain how these phenomena arise, and some of the things that can be done in response.
November 17, 2022
The Tracy-Widom distribution has found broad use in statistical theory and application. We first review some of this, focusing first on Principal Components Analysis and the `spiked model`. When the spike signal is below the Baik-Ben Arous-Peche threshold, likelihood ratio tests for presence of a spike are more efficient than the largest eigenvalue in many settings of multivariate statistics. In recent work in a spiked Wigner model with Egor Klochkov, Alexei Onatski and Damian Pavlyshyn, we study the likelihood ratio test in the transition zone around the BBP threshold, making use of a connection with the spherical Sherrington-Kirkpatrick model and establishing a conjecture of Baik and Lee.
November 18, 2022
Matt Wand and colleagues have recently shown that the machine learning technique of expectation propagation (EP) yields state of the art estimation of parameters in generalized linear mixed models. We review this work before asking: are the EP estimators asymptotically efficient? The problem becomes one of defining an appropriate objective function that captures the EP iteration and approximates maximum likelihood well enough to inherit its efficiency. Joint work with the late Peter Hall, Song Mei, and Matt Wand.
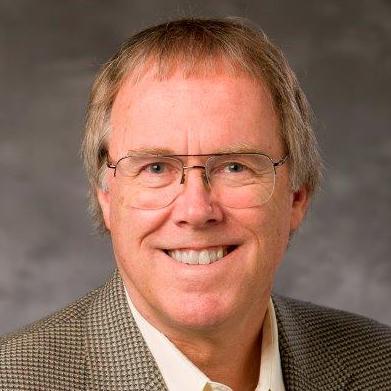
Jim Berger
Duke University
Berger received his Ph.D. degree in mathematics from Cornell University in 1974. He was a faculty member in the Department of Statistics at Purdue University until 1997, at which time he moved to the Institute of Statistics and Decision Sciences (now the Department of Statistical Science) at Duke University, where he is currently the Arts and Sciences Professor of Statistics. He was the founding director of the Statistical and Applied Mathematical Sciences Institute, serving from 2002-2010.
Berger was president of the Institute of Mathematical Statistics during 1995-1996, chair of the Section on Bayesian Statistical Science of the American Statistical Association in 1995, and president of the International Society for Bayesian Analysis during 2004. He has been involved with numerous editorial activities, including co-editorship of the Annals of Statistics during the period 1998-2000 and being a founding editor of the Journal on Uncertainty Quantification, serving from 2012-2015.
Among the awards and honors Berger has received are Guggenheim and Sloan Fellowships, the COPSS President's Award in 1985, the Sigma Xi Research Award at Purdue University for contribution of the year to science in 1993, the COPSS Fisher Lecturer in 2001, the Wald Lecturer of the IMS in 2007 and the Wilks Award from the ASA in 2015. He was elected as foreign member of the Spanish Real Academia de Ciencias in 2002, elected to the USA National Academy of Sciences in 2003, was awarded an honorary Doctor of Science degree from Purdue University in 2004, and became an Honorary Professor at East China Normal University in 2011.
Berger's research has primarily been in Bayesian statistics, foundations of statistics, statistical decision theory, simulation, model selection, and various interdisciplinary areas of science and industry, including astronomy, geophysics, medicine, and validation of complex computer models. He has supervised 36 Ph.D. dissertations, published over 190 papers and has written or edited 16 books or special volumes.
September 8, 2021
Often computer models yield massive output; e.g., a weather model will yield the predicted temperature over a huge grid of points in space and time. Emulation of a computer model is the process of finding an approximation to the computer model that is much faster to run than the computer model itself (which can often take hours or days for a single run). Many successful emulation approaches are statistical in nature. We discuss one such approach – the construction of independent parallel emulators at each grid point – with the emulators being developed through Gaussian processes. The computational simplicity with which this approach can be implemented will be highlighted and the surprising fact that one can ignore spatial structure in the massive output will be explained. All results will be illustrated with a computer model of volcanic pyroclastic flow, the goal being the prediction of hazard probabilities near active volcanoes.
September 9, 2021
The majority of statisticians and scientists who use statistics declare themselves to be frequentists, but they typically mean very different things by this declaration. The purpose of this talk is to highlight the major different types of frequentists and to indicate which are compatible with Bayesianism and which are not. The focus is on evaluating common statistical procedures from the different perspectives, primarily from three unconditional frequentist perspectives.
September 10, 2021
The majority of statisticians and scientists who use statistics declare themselves to be frequentists, but they typically mean very different things by this declaration. This talk is a continuation of Talk 2 on the subject, with the focus shifting to the conditional frequentist perspective, rather than the unconditional frequentist perspective; it is not necessary to have been at the previous talk to follow this one. Larry Brown’s significant work in this area will be highlighted.
Student Workshops
* Winners of Brown best student paper award
Sample splitting for valid hypothesis selection and powerful testing in matched observational studies
Observational studies are valuable tools for inferring causal effects in the absence of controlled 20 experiments. However, these studies may be biased due to the presence of some relevant, unmeasured set of covariates. One approach to mitigate this concern is to identify hypotheses likely to be more resilient to hidden biases by splitting the data into a planning sample for designing the study and an analysis sample for making inferences. We devise a flexible method for selecting hypotheses in the planning sample when an unknown number of outcomes are affected by 25 the treatment, allowing researchers to gain the benefits of exploratory analysis and still conduct powerful inference under concerns of unmeasured confounding. We run extensive simulations that demonstrate pronounced benefits in terms of detection power, especially at higher levels of allowance for unmeasured confounding. Finally, we demonstrate our method in an observational study of the multi-dimensional impacts of a devastating flood in Bangladesh.
Optimal Federated Learning for Nonparametric Regression with Heterogeneous Distributed Differential Privacy Constraints
Denoising diffusion probabilistic models are optimally adaptive to unknown low dimensionality
The denoising diffusion probabilistic model (DDPM) has emerged as a mainstream generative model in generative AI. While sharp convergence guarantees have been established for the DDPM, the iteration complexity is, in general, proportional to the ambient data dimension, resulting in overly conservative theory that fails to explain its practical efficiency. This has motivated the recent work Li and Yan (2024a) to investigate how the DDPM can achieve sampling speed-ups through automatic exploitation of intrinsic low dimensionality of data. We strengthen this prior work by demonstrating, in some sense, optimal adaptivity to unknown low dimensionality. For a broad class of data distributions with intrinsic dimension k, we prove that the iteration complexity of the DDPM scales nearly linearly with k, which is optimal when using KL divergence to measure distributional discrepancy. Our theory is established based on a key observation: the DDPM update rule is equivalent to running a suitably parameterized SDE upon discretization, where the nonlinear component of the drift term is intrinsically low-dimensional.
Boosting e-BH via conditional calibration
The e-BH procedure is an e-value-based multiple testing procedure that provably controls the false discovery rate (FDR) under any dependence structure between the e-values. Despite this appealing theoretical FDR control guarantee, the e-BH procedure often suffers from low power in practice. In this paper, we propose a general framework that boosts the power of e-BH without sacrificing its FDR control under arbitrary dependence. This is achieved by the technique of conditional calibration, where we take as input the e-values and calibrate them to be a set of “boosted e-values” that are guaranteed to be no less—and are often more—powerful than the original ones. Our general framework is explicitly instantiated in three classes of multiple testing problems: (1) testing under parametric models, (2) conditional independence testing under the model-X setting, and (3) model-free conformalized selection. Extensive numerical experiments show that our proposed method significantly improves the power of e-BH while continuing to control the FDR. We also demonstrate the effectiveness of our method through an application to an observational study dataset for identifying individuals whose counterfactuals satisfy certain properties.
On self-training of summary data with genetic applications
Prediction model training is often hindered by limited access to individual-level data due to privacy concerns and logistical challenges, particularly in genetic research. Resampling-based self-training” presents a promising approach for building prediction models using only summary-level data. This method leverages summary statistics to sample pseudo datasets for model training and parameter optimization, allowing for model development without individual level data. In this paper, we use random matrix theory to establish the statistical properties of self-training algorithms for high-dimensional summary data. Interestingly, we demonstrate that, within a class of linear estimators, resampled pseudo-training/validation datasets can achieve the same asymptotic predictive accuracy as conventional training methods using individual level training/validation datasets. These results suggest that self-training with summary data incurs no additional cost in prediction accuracy, while offering significant practical convenience. Furthermore, we extend our analysis to show that the self-training framework maintains this no cost advantage when combining multiple methods (e.g., in ensemble learning) or when jointly training on data from different distributions (e.g., in multi-ancestry genetic data training). We numerically evaluate our results through extensive simulations. Our study highlights the potential= of resampling-based self-training to advance genetic risk prediction and other fields that make summary data publicly available.
* Winners of Brown best student paper award
Higher Order Graphon Theory
In recent years, exchangeable random graphs have emerged as the mainstay of statistical network analysis. Graphons, central objects in graph limit theory, provide a natural way to sample exchangeable random graphs. It is well known that network moments (motif/subgraph counts) identify a graphon (up to an isomorphism). Hence, understanding the sampling distribution of the subgraph counts in random graphs sampled from a graphon is a pivotal problem in nonparametric network inference. In this work, we derive the joint asymptotic distribution of any finite collection of network moments in random graphs sampled from a graphon, including both the non-degenerate case (with a Gaussian distribution) and the degenerate case (with both Gaussian and non-Gaussian components). Furthermore, we develop a novel multiplier bootstrap for graphons that consistently approximates the limiting distribution of the network moments and uses it to construct joint confidence sets for any finite collection of motif densities. To illustrate the broad scope of our results, we also consider the problem of detecting global structure and propose a consistent test for this problem, invoking celebrated results on quasirandom graphs.
Accelerating Convergence of Score-Based Diffusion Models, Provably
[ Proceedings of Machine Learning Research ] [ ArXiv ]
Score-based diffusion models, while achieving remarkable empirical performance, often suffer from low sampling speed, due to extensive function evaluations needed during the sampling phase. Despite a flurry of recent activities towards speeding up diffusion generative modeling in practice, theoretical underpinnings for acceleration techniques remain severely limited. In this paper, we design novel training-free algorithms to accelerate popular deterministic (i.e., DDIM) and stochastic (i.e., DDPM) samplers. Our accelerated deterministic sampler converges at a rate O(1/T^2) with T the number of steps, improving upon the O(1/T) rate for the DDIM sampler; and our accelerated stochastic sampler converges at a rate O(1/T), outperforming the rate O(1/sqrt(T)) for the DDPM sampler. The design of our algorithms leverages insights from higher-order approximation, and shares similar intuitions as popular high-order ODE solvers like the DPMSolver-2. Our theory accommodates l_2-accurate score estimates, and does not require log-concavity or smoothness on the target distribution.
Transfer Learning for Functional Mean Estimation: Phase Transition and Adaptive Algorithms
[ Annals of Statistics ] [ ArXiv ]
This paper studies transfer learning for estimating the mean of random functions based on discretely sampled data, where, in addition to observations from the target distribution, auxiliary samples from similar but distinct source distributions are available. The paper considers both common and independent designs and establishes the minimax rates of convergence for both designs. The results reveal an interesting phase transition phenomenon under the two designs and demonstrate the benefits of utilizing the source samples in the low sampling frequency regime. For practical applications, this paper proposes novel data-driven adaptive algorithms that attain the optimal rates of convergence within a logarithmic factor simultaneously over a large collection of parameter spaces. The theoretical findings are complemented by a simulation study that further supports the effectiveness of the proposed algorithms.
Reconciling Model-X and Doubly Robust Approaches to Conditional Independence Testing
[ Annals of Statistics ] [ ArXiv ]
Model-X approaches to testing conditional independence between a predictor and an outcome variable given a vector of covariates usually assume exact knowledge of the conditional distribution of the predictor given the covariates. Nevertheless, model-X methodologies are often deployed with this conditional distribution learned in sample. In this talk, I will present a comprehensive investigation of the consequences of this choice through the lens of the distilled conditional randomization test (dCRT). I will provide a sufficient doubly robust condition for the dCRT to be protected against Type-I error inflation and this motivates a comparison to the generalized covariance measure (GCM) test, another doubly robust conditional independence test. Interestingly, these two tests are asymptotically equivalent, and semiparametric efficiency theory further unveils that the GCM test is optimal against generalized partially linear alternatives. I will comprehensively compare the finite-sample performance between the GCM and the dCRT and present a simple yet useful approach to drastically improve the finite-sample Type-I error control.
Unbiased Watermark for Large Language Models via Importance Sampling
Watermarking language models enables the detection of text generated by these models, offering a crucial tool for distinguishing between human and machine-generated content, thereby enhancing the integrity and trustworthiness of digital communication. We introduce an unbiased version of the watermarking scheme based on the idea of a green/red partition of the token set, by effectively integrating importance sampling into the decoding process. Our theoretical analysis establishes the unbiasedness and asymptotic detection power of this scheme. Experimental results confirm that, compared to previous methods, our technique more effectively preserves the generation distribution while maintaining competitive detection effectiveness under pseudorandomness. Our findings offer a promising direction for watermarking in language models, balancing the need for detectability with minimal impact on text quality.
* Winners of Brown best student paper award
Stochastic Continuum-Armed Bandits with Additive Models: Minimax Regrets and Adaptive Algorithm
We consider d-dimensional stochastic continuum-armed bandits with the expected reward function being additive β-Hölder with sparsity s for 0 < β < ∞ and 1 ≤ s ≤ d. The rate of convergence for the minimax regret is established where T is the number of rounds. In particular, the minimax regret does not depend on d and is linear in s. A novel algorithm is proposed and is shown to be rate-optimal, up to a logarithmic factor of T. The problem of adaptivity is also studied. A lower bound on the cost of adaptation to the smoothness is obtained and the result implies that adaptation for free is impossible in general without further structural assumptions. We then consider adaptive additive SCAB under an additional self-similarity assumption. An adaptive procedure is constructed and is shown to simultaneously achieve the minimax regret for a range of smoothness levels. This is joint work with Dr. Tony Cai.
Optimal Refinement of Strata to Balance Covariates
[ Biometrics ]
What is the best way to split or refine one stratum into two strata if the goal is to maximally reduce the within-stratum imbalance in many covariates? We formulate this problem as an integer program and show how to nearly solve it by randomized rounding of a linear program. A linear program may assign a fraction of a person to one refined stratum and the remainder to the other stratum. Randomized rounding views fractional people as probabilities, assigning intact people to strata using biased coins. Randomized rounding of a linear program is a well-studied theoretical technique for approximating the optimal solution of classes of insoluble (i.e., NP-hard) but amenable integer programs. When the number of people in a stratum is large relative to the number of covariates, we prove the following new results: (i) randomized rounding to split a stratum does very little randomizing, so it closely resembles the unusable linear programming solution that splits intact people, (ii) the unusable linear programming solution and the randomly rounded solution place lower and upper bounds on the unattainable integer programming solution, and because of (i) these bounds are often close, thereby ratifying the usable randomly rounded solution. We illustrate using an observational study that balanced many covariates by forming 1008 matched pairs composed of 2 × 1008 = 2016 patients selected from 5735 using a propensity score. Instead, we: (i) form five strata using the propensity score, (ii) refine them into ten strata, (iii) obtain excellent covariate balance, (iv) retain all 5735 patients. An R package optrefine implements the method. This is joint work with Dr. Dylan Small and Dr. Paul Rosenbaum.
Doubly Robust Prediction under Covariate Shift
Conformal prediction has received tremendous attention in recent years offering new solutions to problems in missing data and causal inference. This work moves beyond the usual assumption that the data are iid (or changeable) and considers the problem of obtaining distribution-free prediction regions accounting for a shift in the distribution of the covariates between the training and test data. Under a standard covariate shift assumption analogous to the missing at random assumption, we propose a general framework to construct well-calibrated prediction regions for the unobserved outcome in the test sample. Our approach is based on the efficient influence function for the quantile of the unobserved outcome in the test population combined with an arbitrary machine learning prediction algorithm, without compromising asymptotic coverage and it is established that the resulting prediction sets eventually attain nominal coverage in large samples. I will further show that in the setting of covariate shift, it is impossible to have any informative result without other knowledge, e.g. how much the shift is, and therefore, asymptotic results are as good as one can hope for. We leverage semiparametric theory so that correct coverage would be guaranteed if either the propensity score or the conditional distribution of the response is estimated sufficiently well, hence 'doubly robust.' I will also discuss how this would extend to constructing doubly robust prediction sets of individual treatment effects, and how aggregation of different algorithms could be leveraged for optimal prediction set. This is joint work with Dr. Arun Kuchibhotla and Dr. Eric Tchetgen Tchetgen.
* Winners of Brown best student paper award
Social Distancing and Covid-19: Randomization Inference for a Structured Dose-Response Relationship
[ Annals of Applied Statistics ] [ ArXiv ]
Social distancing is widely acknowledged as an effective public health policy combating the novel coronavirus. But extreme forms of social distancing like isolation and quarantine have costs and it is not clear how much social distancing is needed to achieve public health effects. In this article, we develop a design-based framework to test the causal null hypothesis and make inference about the dose-response relationship between reduction in social mobility and COVID-19 related public health outcomes. We first discuss how to embed observational data with a time-independent, continuous treatment dose into an approximate randomized experiment, and develop a randomization-based procedure that tests if a structured dose-response relationship fits the data. We then generalize the design and testing procedure to accommodate a time-dependent treatment dose in a longitudinal setting. Finally, we apply the proposed design and testing procedures to investigate the effect of social distancing during the phased reopening in the United States on public health outcomes using data compiled from sources including Unacast™, the United States Census Bureau, and the County Health Rankings and Roadmaps Program. We rejected a primary analysis null hypothesis that stated the social distancing from April 27, 2020, to June 28, 2020, had no effect on the COVID-19-related death toll from June 29, 2020, to August 2, 2020 (p-value < 0.001), and found that it took more reduction in mobility to prevent exponential growth in case numbers for non-rural counties compared to rural counties.
The Cost of Privacy in Generalized Linear Models: Algorithms and Minimax Lower Bounds
[ Annals of Statistics ] [ ArXiv ]
In this paper, we propose differentially private algorithms for parameter estimation in both low-dimensional and high-dimensional sparse generalized linear models (GLMs) by constructing private versions of projected gradient descent. We show that the proposed algorithms are nearly rate-optimal by characterizing their statistical performance and establishing privacy-constrained minimax lower bounds for GLMs. The lower bounds are obtained via a novel technique, which is based on Stein’s Lemma and generalizes the tracing attack technique for privacy-constrained lower bounds. This lower bound argument can be of independent interest as it is applicable to general parametric models. Simulated and real data experiments are conducted to demonstrate the numerical performance of our algorithms.
Multiaccurate Proxies for Downstream Fairness
[ 2022 ACM Conference on Fairness, Accountability, and Transparency ] [ ArXiv ]
We study the problem of training a model that must obey demographic fairness conditions when the sensitive features are not available at training time — in other words, how can we train a model to be fair by race when we don’t have data about race? We adopt a fairness pipeline perspective, in which an “upstream” learner that does have access to the sensitive features will learn a proxy model for these features from the other attributes. The goal of the proxy is to allow a general “downstream” learner — with minimal assumptions on their prediction task — to be able to use the proxy to train a model that is fair with respect to the true sensitive features. We show that obeying multiaccuracy constraints with respect to the downstream model class suffices for this purpose, and provide sample- and oracle efficient algorithms and generalization bounds for learning such proxies. In general, multiaccuracy can be much easier to satisfy than classification accuracy, and can be satisfied even when the sensitive features are hard to predict.
Increasing Power for Observational Studies of Aberrant Response: An Adaptive Approach
In many observational studies, the interest is in the effect of treatment on bad, aberrant outcomes rather than the average outcome. For such settings, the traditional approach is to define a dichotomous outcome indicating aberration from a continuous score and use the Mantel-Haenszel test with matched data. For example, studies of determinants of poor child growth use the World Health Organization’s definition of child stunting being height-for-age z-score ≤ -2. The traditional approach may lose power because it discards potentially useful information about the severity of aberration. We develop an adaptive approach that makes use of this information and asymptotically dominates the traditional approach. We develop our approach in two parts. First, we develop an aberrant rank approach in matched observational studies and prove a novel design sensitivity formula enabling its asymptotic comparison with the Mantel-Haenszel test under various settings. Second, we develop a new, general adaptive approach, the two-stage programming method, and use it to adaptively combine the aberrant rank test and the Mantel-Haenszel test. We apply our approach to a study of the effect of teenage pregnancy on stunting.